Predictive analytics and machine learning usually come together like peanut butter and jam. They create an intelligent tech background for uncovering hidden insights and opportunities. So, it’s no surprise that they are often used interchangeably.
Both analytic strategies yield unprecedented possibilities for businesses. However, each provides a different extent of capabilities and is used for slightly different areas. At least 37% of executives don’t know the difference or the benefits they bring to the company. So which one should your company use, and how do we adopt it?
Today, we’ll find out about core differences between predictive analytics vs machine learning, how they’re connected, and why they matter.
A Few Words about Machine Learning
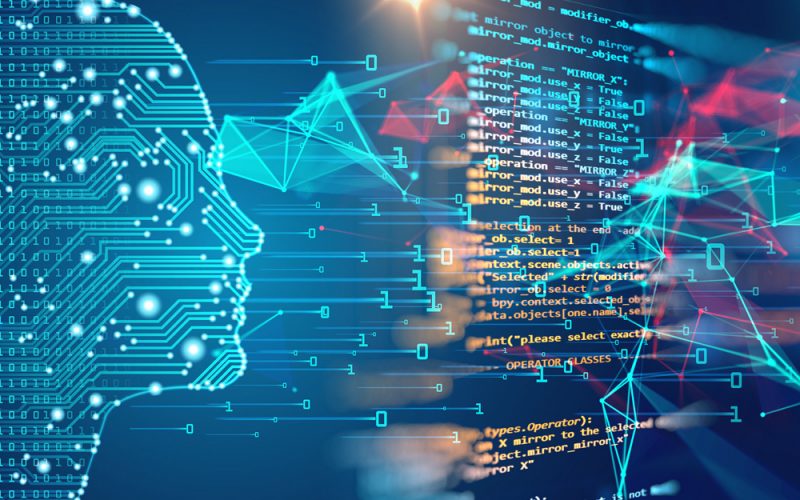
Let’s start with the basics. Machine learning is a branch of artificial intelligence. In particular, it provides the ground for analyzing data that allows a system to learn independently by solving an array of similar problems. Therefore, it is a set of mathematical, statistical, and computational methods for developing algorithms that allow a machine to uncover patterns on its own.
Machine learning comes in different flavors based on the training data:
- Supervised learning – is a type of machine learning that relies on the use of labeled datasets to train algorithms. It means that it contains data tagged with the correct answer. After that, the machine is fed another set of data so that the algorithm analyses the new input based on the previously labeled data.
- Unsupervised learning – is a type of machine learning that relies on the use of unlabeled datasets to train algorithms. Hence, ML algorithms must first self-discover any naturally occurring patterns in that training data set.
- Reinforcement learning – is a feedback-based ML technique that is based on the trial and error principle to come up with a solution to the problem.
Why Use Machine Learning For Your Business
Machine learning opens up a wealth of unrivaled possibilities for computers to solve tasks previously performed by humans. Moreover, training data lays the ground for accurate predictions. Finally, machine learning is one of the most common uses of artificial intelligence in modern business.
Thus, it contributes to the global AI market which will snowball in the next few years, climbing to a $190.61 billion market value in 2025.
Here’s how global companies are tapping into the smart capabilities of machine learning.
1. Predictive maintenance
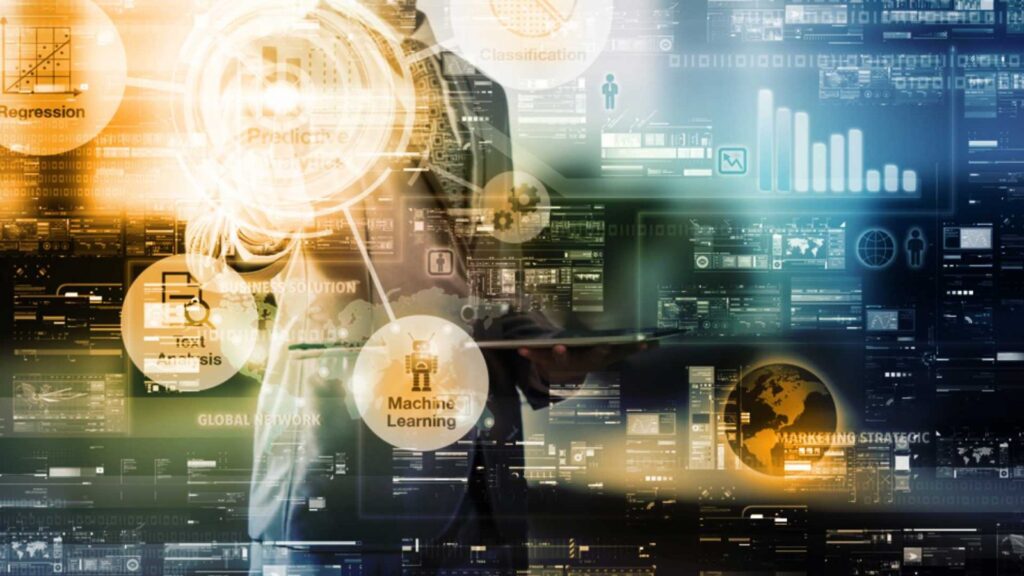
In manufacturing, ML improves asset operation and eliminates costly outages by applying computation to large amounts of sensor data. Anomaly detection also helps manufacturers reduce machine and equipment downtime – from assembly lines to jet engines.
2. Rapid data processing and real-time forecasting
With a growing amount of Big data, businesses need an effective and fast way to process and analyze it. ML algorithms allow companies to transform information into knowledge in real-time, thus amplifying business decision-making. Machine learning can also analyze market demands and potential changes.
3. Customer behavior
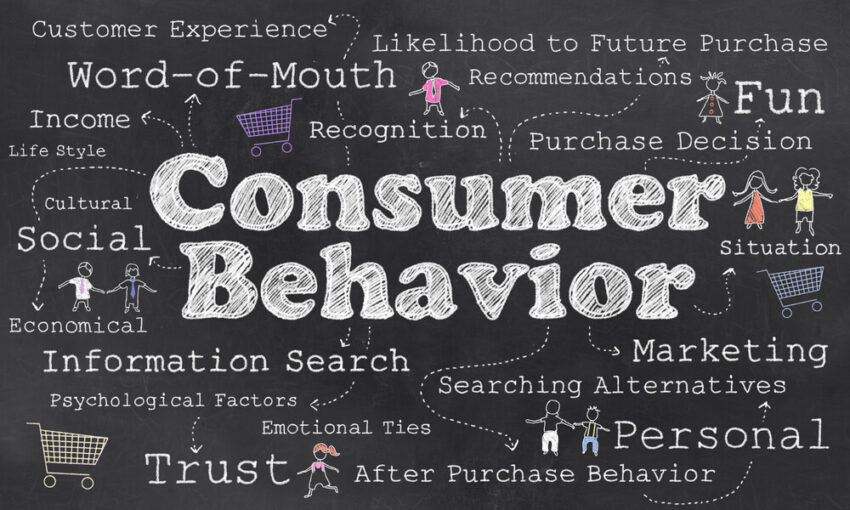
ML algorithms also help predict customer behavior and fine-tune marketing efforts. This application includes predictive and prescriptive data in order to expand the consumer base or provide more personalized services. By analyzing their clients’ behavior, buying patterns, and shopping history, companies personalize product recommendations according to the preferences of individual customers.
4. Improved cybersecurity
Cybersecurity and network intrusions haunt global businesses and result in significant losses. Machine learning is used to bolster internal security measures and shield from cyber attacks. Fraud and anomaly detection, spam filtering, and data-leak prevention are just some examples of ML in cybersecurity.
These are just a sliver of all the smart capabilities of machine learning. Thanks to its analytical nature, it is a salient part of enterprise systems linked with various fields.
A Few Words about Predictive Analytics
As its name implies, predictive analytics identifies the likelihood of future events based on analysis of accumulated data. This discipline inherits the best from mathematical statistics, modeling, machine learning, and other areas of Data Science.
Here’s what the step-by-step process of predictive analytics looks like:
1. Project definition/requirement collection
The analysis starts with identifying problems to be solved with predictive analytics. The client articulates the kind of predictions they want from the system.
2. Data collection
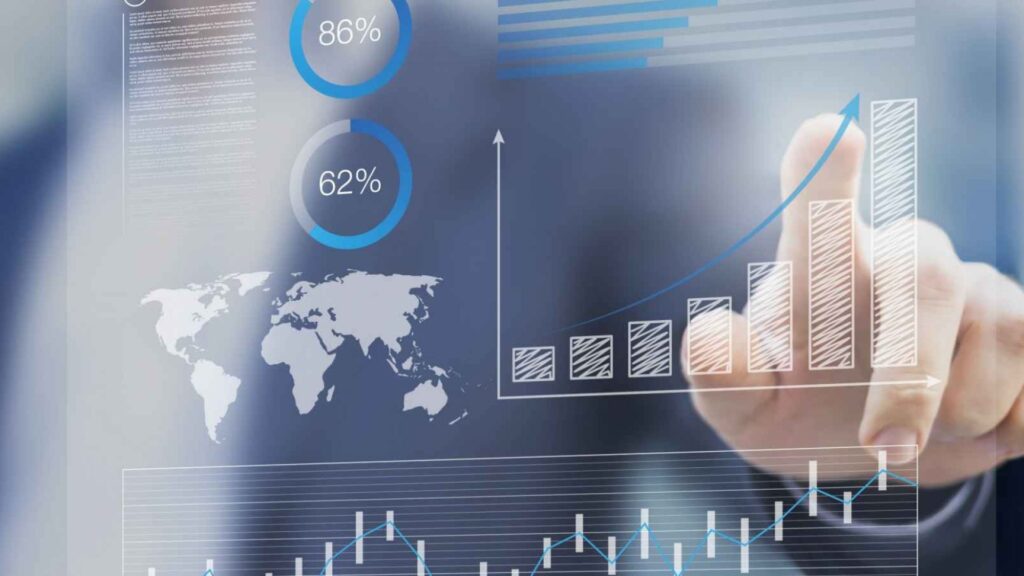
Data is the basis of any statistical analysis, a technique that includes machine learning. Thus, you need a great amount of data to draw conclusions. Hence, the volume and quality of data are the two cornerstones for successful analysis.
3. Exploratory data analysis
The volumes of raw data alone are insufficient for predictive analytics. Identifying patterns in today’s volumes of data requires an adequate approach. Artificial intelligence technologies help keep the volumes of raw data at bay by revealing hidden relationships.
4. Predictive modeling
Generating insights is the next and final step. It involves building a mathematical predictive model to solve the problem at hand. Machine learning acts as a driving force for generating predictions.
When Use Predictive Analytics for Your Business?
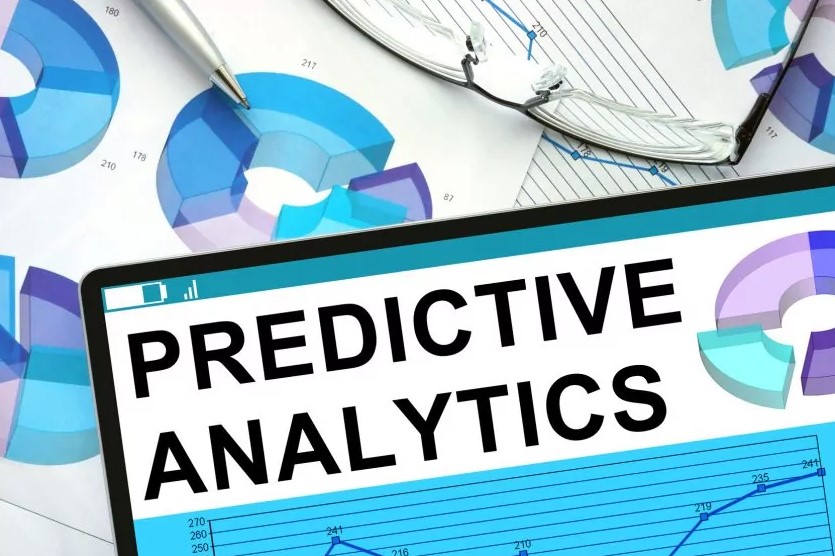
Traditional analytics methods are relevant for long-term forecasting, as well as for products with a rich sales history and established demand.
On the contrary, predictive analytics works wonders with undefined variables. Therefore, this approach works best for short-term and medium-term forecasts. These may include new products, seasonal items, or products with a short life cycle.
Besides, predictive analytics is widely used in the following fields:
- Customer segmentation – dividing customers into groups based on similar needs to offer them relevant products and services.
- Optimized supply chains – predict delivery windows and shipping times by analyzing real-time data.
- Equipment maintenance – avoiding unscheduled and accidental breakdowns by predicting the likelihood of malfunctions.
- Churn prevention – averting brand switching by identifying signs of dissatisfaction among your customers
- Credit risk and fraud prevention – learning potential areas of risk from various data points.
By this time, you must’ve already guessed the kind of relationships between analytics and machine learning. But there’s more to that.
Predictive Analytics and Machine Learning: What’s the difference?
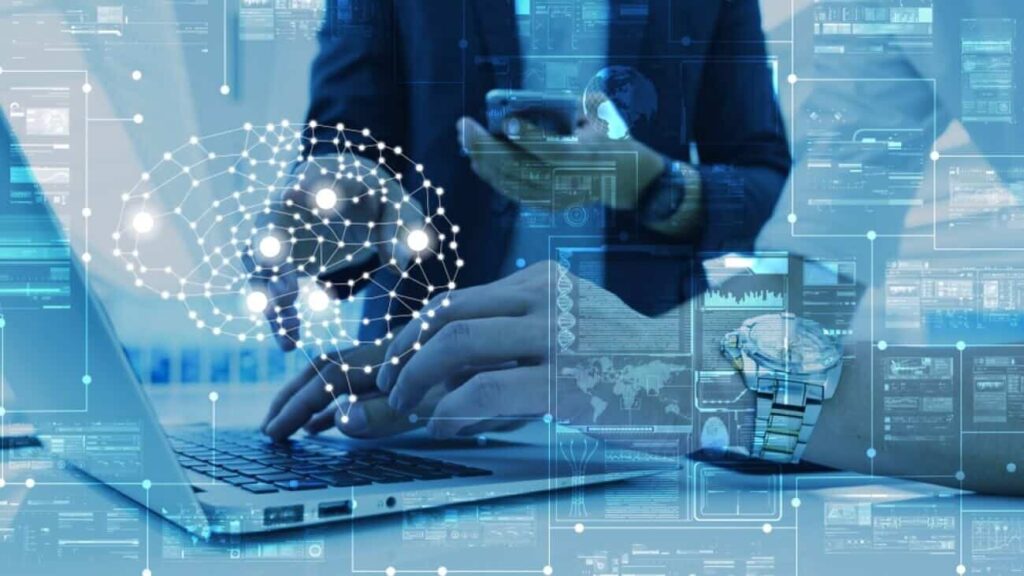
At first sight, both look almost identical, sharing a number of fundamental similarities, including:
- Both predictive analytics and machine learning look into hidden relationships to produce reliable outcomes.
- A comprehensive amount of data is a mandate for both technologies.
- Predictive modeling is usually the overriding objective of both.
Indeed, both disciplines complement each other, thus contributing to a common goal. However, they are not synonyms.
First of all, machine learning relies on computer science, while predictive analytics stems from statistics. Secondly, machine learning requires a lot of coding compared to predictive analytics. Also, PA systems can rely on machine-learning algorithms, while the latter doesn’t need analytics to yield an accurate result.
These differences may appear little, but they are significant enough to separate the two fields. Although machine learning can be used to create a predictive data model, it will be useless without a thorough analytics approach. Furthermore, machine learning is only one of many technologies that can be utilized to create predictive models.
The Bottom Line
In the data-dominated business world, it is crucial to stay on top of that data. Machine learning and predictive analytics are the cornerstone technologies that assist businesses on the way to digital transformation and holistic decision-making.
Although the two share significant similarities, machine learning and analytics are different branches that help yield similar results. Thus, if your company looks for at-scale automation, ML-powered software is a more suitable option. On the contrary, if you’re focused on analytical capabilities, predictive analytics will take advantage of future trends and, at the same time, avert challenges before they cripple your operations.